By Savannah Hunter and Pamela Reynolds
How can college students prepare to enter today’s diverse, data-driven workforce? As instructors, how can we create opportunities for our students to acquire necessary skills to grow professionally and personally towards their future technical careers? Investing in opportunities for experiential learning may afford answers to both questions. Experiential learning, a student-focused approach uniting research and career skills training with traditional classroom instruction of theory and concepts, helps students grow professionally and personally, preparing them for their future careers. University-organized data challenges, particularly those taking place in a virtual space, provide opportunities for experiential learning that work to break down traditional barriers in STEM education promoting diversity and equitable access to these experiences.
Date | Event |
---|---|
September 14 | Virtual Kick-Off and Team Match-making Session |
September 16 | Mentor Q&A Session 1 |
September 18 | Mentor Q&A Session 2 |
September 21 | DataLab Technical Drop-in Office Hours 1 |
September 28 | DataLab Technical Drop-in Office Hours 2 |
September 30 | DataLab Technical Drop-in Office Hours 3 |
October 5-6 | Virtual Showcase |
October 21 | Public Finale Webinar |
Learning as an active experience
‘Experiential learning’ is an educational philosophy with roots in the work of John Dewey, Kurt Lewin, Jean Piaget, Paulo Freire, and others in which learners generate their own knowledge through experiencing, reflecting, thinking, and acting on a topic (Kolb and Kolb 2005; Pedaste 2015). Experiential learning opportunities in higher education often include for credit internships, courses with a service-learning component, independent or collaborative research projects with faculty, and study abroad (Moore 2010). These opportunities allow learners to apply, reinforce, and generate new knowledge in real-world settings. Students may learn more when experiential learning is incorporated into their education (Pedaste 2015), gaining valuable practical experience that serves them in their personal and career development (Holtzman and Menning 2015; Moore 2010; Van Wart et al. 2020).
Opportunities for hands-on learning are becoming increasingly critical for entering data-driven careers, which require technical proficiencies as well as mastery of concepts and theory. Experiential learning can help graduates gain a leg up in the job market by facilitating the development of technical portfolios, which are increasingly key for demonstrating computational knowledge and abilities (Ring et al. 2017). While embedding experiential learning in long-format instruction, such as traditional courses, is transformative for knowledge acquisition, integrating hands-on learning in virtual and short-format engagements can further promote learner diversity and more equitable access (Strait et al. 2015; Wright 2000).
Data challenges, sometimes also called hackathons, codefests or datafests, are an example of opportunities for short-format experiential learning in technical spaces (Gama et al. 2018). In academic contexts, these activities typically occur outside the traditional classroom and allow for a wider diversity of participant backgrounds, domain training, and stage of academic career. Prizes and certificates are awarded to learners in lieu of grades and course credit. As a case study to explore how intentionally organized data challenges can serve as opportunities for short-format experiential learning, below we discuss our experience organizing a month-long data challenge sponsored by the UC Davis DataLab: Data Science and Informatics department and the Scholars Strategy Network that coincided with the November 2020 election.
The CA Election 2020 Data Challenge: experiential learning with a focus on data literacy and civic engagement

The CA Election 2020 Data Challenge charged participants to create a reproducible research project culminating in a data visualization examining a specific issue relating to one of the California ballot initiatives. The Challenge created a unique space for participants to develop the skills to educate themselves and others on pressing social issues facing us as voters while honing their technical skills. The Challenge goals included fostering critical skills of data literacy, data visualization, reproducible research, teamwork, and civic engagement.
The Challenge engaged learners from diverse academic and personal backgrounds. Engaging 113 participants hailing from over 30 disciplines including statistics, the life sciences, social sciences, and computer science, participants were evenly split between undergraduate and graduate students. The Challenge also garnered interest and engagement from high schoolers, alumni, post docs, professionals, and university staff. A majority of the participants who submitted Challenge projects identified as women (around 60%). While we did not explicitly collect ethnoracial information from participants, half of the participants who responded to an anonymous post-challenge feedback survey indicated that they did not identify as Caucasian/white.
Engaging with the research process
Exposure to and engagement in research is a goal of the University of California, and the activities of this experiential learning case study were organized parallel to the pursuit of the scientific method (à la Pedaste 2015). Student teams followed their curiosity about an issue on the ballot (‘Orientation’), developed a research question to explore that issue (‘Conceptualization’), explored and located publicly available data to address their research question (‘Investigation’), drew conclusions based on the data (‘Conclusion’), and finally communicated those conclusions to others and reflected on what they learned by submitting a video presentation of their project to an asynchronous, Virtual Showcase (‘Discussion’). A panel of judges reviewed projects selecting three teams whose exemplary projects were accessible to a broad audience, demonstrated innovative approaches, and asked critical research questions. These three winning teams, self-named Catch-22, install.packages(“tidywitches”), and MissDemeanors, presented at a public Finale Webinar alongside invited keynote speakers from the academic and civic community.
Developing technical skills, addressing pressing social issues
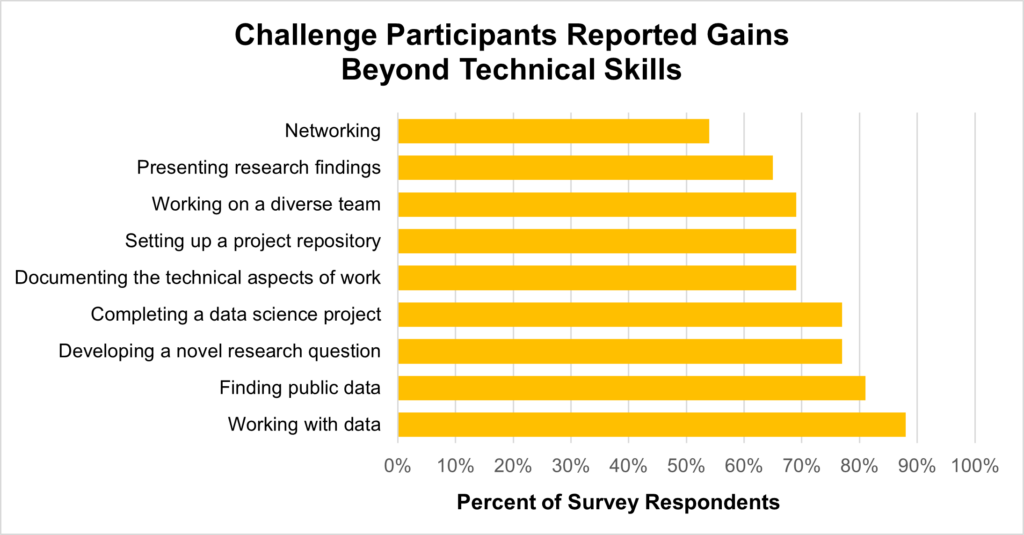
Experiential learning encourages students to incorporate new ideas into previous understandings, question or modify existing knowledge, and create new knowledge and skills (Kolb 2005). Challenge participant respondents of the anonymous feedback survey reported strong gains in terms of learning about one or more ballot initiatives (88%), strategies for finding publicly available data (69%), teamwork skills (62%), a new data visualization technique (62%), and new tool or technology (62%). Additionally, a majority of participants said their confidence improved working with data, finding publicly available data, developing a research question, completing a data science project, documenting their work, setting up a project repository, working with a diverse team, presenting research findings and networking.
Additionally, experiential learning presents students with the opportunity to take charge of their learning and become agents of social change (Breunig 2005). While a goal of the Challenge was to help students graduate with practical skills and experience, its design focus on civic projects sought to encourage them to leverage these skills to become active and informed voters and citizens. The Challenge created a unique space for participants to develop the skills to educate themselves and others on pressing social issues facing us as voters while honing their technical skills.
Making space for learning
Creating an environment for successful experiential learning takes intention. Without close attention to the structure and organization of a challenge, the focus on personal skills development, collaborative learning, inclusivity, and creativity can give way under the pressure of competition. While competition during challenges can promote quick solutions, it often comes at the cost to the participants and the program by reducing personal skill development and overall inclusivity and creativity (Richterich 2019). Drawing on Kolb and Kolb (2005)’s framework for creating successful experiential learning spaces, we unpack features of our Challenge that created a successful learning space, and provide recommendations for how data challenges and hackathons can serve as experiential learning models for creating real-world opportunities for students to grow their technical, social, and civic knowledge and skill sets.
1. Make space for learners to take charge of their own learning
We provided specific guidelines on Challenge goals and support structures to participants throughout the Challenge. However, participants took complete ownership over their projects and their own learning. They started this journey by self-organizing into teams. We offered a team match-making session, encouraged participants to fill out a registration form indicating their interest in finding teammates, and created online communication channels (via a private Challenge participant-only Slack workspace) specifically designated for participants to identify teammates. Teams developed their own division of labor and strategy for working with each other.
Participants designed their own research question. Challenge participants were able to pursue the questions that were meaningful and interesting to them. They developed and refined their questions, and identified publicly available data to pursue those questions. While participant-led, this effort didn’t occur in a vacuum. Two facilitated Q&A sessions with domain experts (including research librarians) from across the university and beyond helped support participants in understanding the ballot initiatives, find relevant data, and hone their research questions.
Participants did the work. For this Challenge that meant scraping or otherwise collecting the data, and performing all their own data cleaning, analysis, and visualization. In addition to an overview onboarding presentation that included general help sources, two scheduled drop-in technical office hours sessions were available for the teams to meet with experienced data scientists for help when they got stuck. An online communication “help me” channel (in Slack) designated for the Challenge participants to ask, and give, help to one another facilitated collaboration and innovation across teams.
Participants shared and reflected on their accomplishments, and their struggles. The entire Challenge occurred virtually due to the COVID-19 pandemic. Thus, any sharing of projects also had to occur in virtual space. To best accommodate participation by the diverse suite of participants, teams presented their findings via short 10-minute videos and links to their GitHub project repositories, to a 2-day, asynchronous online showcase. In preparation for this event an optional “check-in” session was held to review the submission criteria, and provide a tutorial of how to document digital research projects. This included reviewing the purpose and contents of a README file, and discussing examples of how repositories can be organized for reproducible research. To align expectations and ensure participants focused on what they learned (versus worry about ancillary aesthetics) templates were provided for both the project README files and presentation slides. Prompts on the slides facilitated both team and individual self-assessment of their learning and overall experience with the Challenge. All participants, judges and community members were encouraged to ask questions directly to the teams during the Showcase, furthering connections and engagement with the domain and technical aspects of the research.
Recommendations: Additional opportunities for feedback, particularly at the mid-point for longer-duration data challenges as well as a pre-submission check-in, would enhance this learning process. Project-focused feedback from the judges for all teams is also recommended to provide a more robust form of summative assessment to the learners.
2. Create a supportive learning community based on belonging and mutual respect
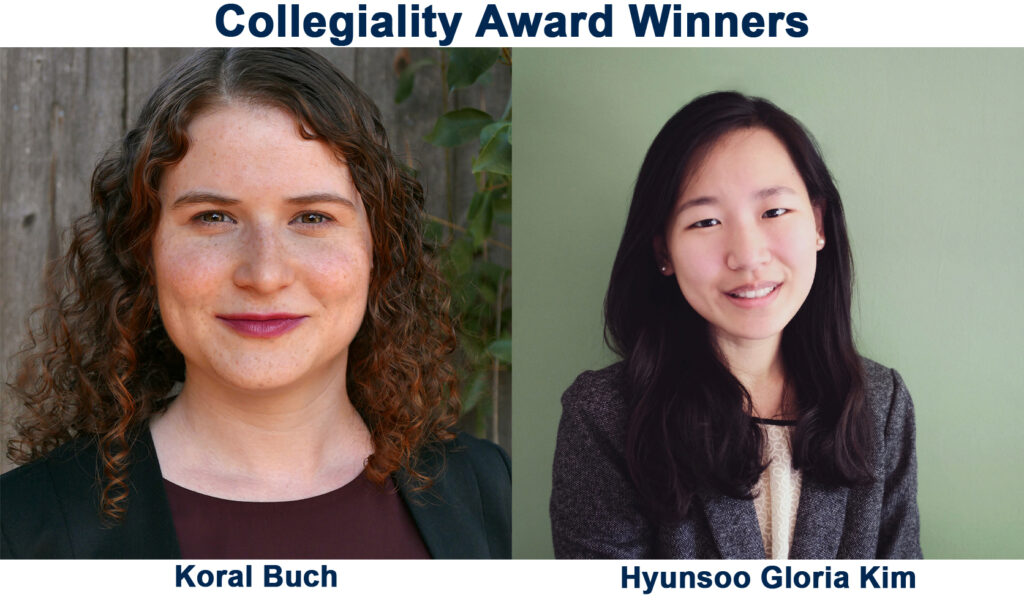
Creating a sense of community online, during a global pandemic, and during a short time frame was an unexpected hurdle the organizers of our Challenge had to address intentionally. Typically, community-building would happen through in-person activities including info sessions and ice breaker team matchmaking events, pizza hack nights, open-door ‘drop in’ help sessions, and other socially driven engagements. For the CA Election 2020 Data Challenge, we had to develop and rely exclusively on virtual engagements to build a supportive community.
The initial virtual onboarding meeting explicitly established expectations for participants and guidelines for the projects, identified communication platforms and how they could give and receive help, and set the stage for a code of conduct for all participants. All of the virtual Challenge activities, from that initial onboarding meeting to the office hours and subsequent check-ins allowed for supportive touch points for participants to interact with organizers, mentors, and each other to receive guidance and support. We encouraged participants to make use of the provided online channels (via Slack) to interact within and across teams, and to support one another. This allowed for a safe and low risk space for participants to ask questions, toss out ideas, share resources, and ultimately post their Challenge projects.
The Challenge organizers and mentors modeled supportive community-driven discourse by frequently engaging with the participants on those platforms, recognizing accomplishments and pain points, suggesting strategies, and helping make connections. At the end of the Challenge, we publicly recognized all participants for their efforts and issued completion certificates to those individuals who participated on teams that submitted a project to the Showcase. We also offered two Collegiality Awards for participants who went above and beyond and selflessly supported competing teams, offered thoughtful feedback on others’ work, and made an effort to foster community during the Challenge.
Recommendations: Create collaborative community guidelines, in addition to a code of conduct, that describes how Data Challenge participants will relate and interact with one another. Create frequent additional informal opportunities for participants, mentors, and organizers, to interact in addition to formal sessions.
3. Meet learners where they are and build on their prior experience
In this Challenge, participants developed their own research question and project goals. Thus, they self-defined their own starting place based on their existing skills, available resources, and interests. We required that all projects be reproducible, and provided tutorials and resources to help them set up repositories on GitHub. While we encouraged participants to use open source software, we did not require them to have prerequisite research, coding, or specific software expertise. The Challenge charged them to create an accessible “data visualization” without setting requirements or standards on what they could produce. Our goal was to allow learners to grow at their own pace and in ways that were meaningful to them and their individual and team learning goals. We also sought to encourage creativity in the design and manifestation of the projects. This helped to meet the two most common participant goals of growing their technical skillset, and contributing to “data science for social good”. Because we wanted to engage individuals from as many domains as possible and to encourage vertically integrated teams, we anticipated individual and team backgrounds in data science to be highly variable. Thus, we did not set prizes for “undergraduate” vs “graduate” student awards.
Recommendations: Considering creating prize categories to recognize participants at different types of contributions and levels of experience. Growth can be difficult to self-assess, and is important to be celebrated in these spaces where learners are often pivoting to acquire and apply new skills.
4. Make space for development of expertise
The Challenge’s month-long time frame and call to develop a research question and a data visualization for a ballot initiative encouraged development of both technical and domain expertise. Participants oriented themselves to a new social issue broadly, but also had to employ research strategies and frameworks to develop a narrow, specific and actionable research question. Participants gained knowledge about their data source(s) and were asked to think about what the data they selected could – and importantly could not – say about their particular research question. Finally, each group developed or honed their expertise in data analysis, data visualization, and teamwork.
Some participants demonstrated their comprehensive understanding of the issue by examining it in a multidimensional way (see Team Dialysis Analysis for an example). Many demonstrated new skills for data analysis and presentation. Particularly, use of newer interactive data visualizations using RShiny (see Team Wobbler Costs for an example) were popular among the teams who also had to learn associated technical and project development skills regarding web servers and hosting.
Recommendations: Provide participants with access to mentors demonstrating a range of expertise including technical knowledge, subject matter expertise, and research design (developing questions, identifying data, etc.). Provide infrastructure as needed to facilitate hosting projects.
5. Make space for learning guided by intrinsic motivation
Shared vision, goals, and passions are key features of successful research teams. While the Challenge awarded monetary prizes to three finalist teams and two collegiality award winners, our participant recruitment efforts focused on the social issues involved (i.e., health care, labor regulation, criminal justice, affirmative action, voting rights, affordable housing, consumer privacy, and tax reform) and the career-development benefit of having a portfolio to demonstrate technical skill sets rather than a specific prize. As such, recruitment efforts focused on existing affiliates, departments, undergraduate and graduate clubs both in the data sciences and proposition-related domain focuses. The monetary prizes (capped at $500 per team) served as a recognition award for their efforts to create robust, reproducible and impactful projects and community engagement rather than the sole incentive for participating in the Challenge.
Life experiences and concerns for the impact of the proposition topics on participants’ lives and the lives of others motivated many projects.
It is clear that participants were intrinsically motivated by the Challenge. During on-boarding many participants mentioned being drawn to the Challenge because they wanted to be a part of a community, expressed a love of working with data, described a passion for or interest in learning more about local civic issues from a data-driven perspective, or a desire to build and demonstrate their skills as part of their professional development. Additionally, during the Virtual Showcase we prompted participants to share their motivation for the specific ballot initiative and/or research question they pursued. Life experiences and concerns for the impact of the proposition topics on participants’ lives and the lives of others motivated many projects. In particular, criminal justice reform (Proposition 17 – Parolee Voting Rights) and affordable housing (Prop 21 – Rental Control), were popular among participants reflecting broader campus and community concerns about these issues.
Recommendations: Select a meaningful focus for the Data Challenge that allows for learners to pursue their goals in a variety of ways. Orient the data challenge advertising to highlight the overarching issue(s) participants will engage with in addition to the skills participants will develop. Provide general guidance and allow participants to select their own topics and approaches for tackling their projects.
6. Make space for both feeling and thinking
Experiential learning that allows space for emotion, allowing students to follow their passions and interests, results in deeper learning and persistence in science (Corwin et al. 2015; Felten et al. 2006). In this Challenge, participants selected their project topics from one of twelve initiatives on the California ballot, allowing their own interests to guide their work. Particularly in light of the politically-charged landscape of 2020, one concern was to foster collegial civic engagement. During the orientation we reinforced that political agendas or, potentially, biased work would not be tolerated, and participants were warned that they would be dismissed from the Challenge if they sought to subvert the primary goals of the Challenge to experience the process of working with data to uncover insights and apply data science to address real-world challenges. We described that the project and data visualization could encompass anything related to the ballot initiative, allowing students to follow their passions, but that the Challenge would not support political agendas. Teams could explore a question related to an argument in favor of or against a proposition, but this framing had to be data-driven, both sides of the issue presented, and the limits of their analysis clearly articulated. The goal was to enable quantitative exploration of a social issue, not to convince anyone how to vote.
Recommendations: Encourage participants to follow their passions grounded in the scientific method to test ideas and improve their fluency of the data, approaches, and perspectives of a complex issue.
7. Make space for acting and reflecting on knowledge
Often students learn theories or skills in the classroom, but have limited opportunities to apply them or reflect on them in ways that are personally meaningful. This Challenge presented an opportunity for participants to act and expand on existing skills learned in formal coursework using real world, often messy public data. Of the participants who responded to a post-Challenge experience survey, one-third stated that the Challenge was their first exposure to research. Additionally, most of the respondents had never previously participated in a Data Challenge or similar event (85%). Allowing participants to develop their own research questions exposed them to the process of doing research, testing their ideas, and exploring their curiosities in a self-directed, hands-on way. For the Virtual Showcase we asked participants to summarize and reflect on what they learned, and describe what they struggled with and might do differently in the future. This process of reflection is one of the most important components of experiential learning as it encourages learners to think about how classroom knowledge translates to real-world settings and encourages the incorporation of new knowledge and experiences (Kolb and Kolb 2005; Moore 2010).
Recommendations: Project outcomes should reinforce learning, encouraging participants to reflect on what they have accomplished. Hosting additional peer and mentor feedback sessions during, and after, the Data Challenge would provide additional opportunities for reflection. Questions on participant surveys can be designed to both help with program assessment as well as reinforce the learners’ self-assessment and reflection.
8. Experiential learning spaces require institutional support
Creating an opportunity for experiential learning takes a team resourced with time and programmatic support. Planning for the Challenge began a year prior to the event and increased in intensity during the few months prior to the Challenge launch. The Challenge required staff and volunteer time in addition to award prizes. This Challenge involved two part-time organizers, a part-time student assistant, part-time communications assistance, 25 volunteer technical and subject matter expert mentors (2-4 hours of work each), and 11 volunteer expert judges (up to 8 hours of work each).
Recommendations: Data Challenges with successful experiential learning require significant institutional support, including staff time and expertise for development and coordination, as well as monetary resources for events and prizes.
Conclusion
To meet today’s diverse learner populations, academic institutions should actively engage in data challenges as opportunities for experiential learning. Experiential learning is an important tool for increasing student learning and preparing students for professional careers in technical and data-driven industries. The 2020 CA Election Data Challenge hosted by UC Davis demonstrates that, even in an all-virtual model, students can achieve strong gains in skills and confidence working on data-intensive research. Intentionally organized data challenges provide critical short-format experiential opportunities that open doors for a diversity of students to build on their learning from the traditional classroom and practice new skills, from teamwork and data literacy to data visualization. Institutional investment in design, coordination, and interdisciplinary engagement is necessary to maximize the impact of data challenges to best prepare our students to grow personally and professionally toward launching their careers in today’s data-driven workplace.
References Cited
- Breunig, Mary. 2005. “Turning Experiential Education and Critical Pedagogy Theory into Praxis.” Journal of Experiential Education 28(2):106–22. doi: 10.1177/105382590502800205.
- Corwin, Lisa A., Mark J. Graham, and Erin L. Dolan. 2015. “Modeling Course-Based Undergraduate Research Experiences: An Agenda for Future Research and Evaluation.” CBE—Life Sciences Education 14(1):es1. doi: 10.1187/cbe.14-10-0167.
- Felten, Peter, Leigh Z. Gilchrist, and Alexa Darby. 2006. “Emotion and Learning: Feeling Our Way Toward a New Theory of Reflection in Service-Learning.” Michigan Journal of Community Service Learning 38–46.
- Gama, Kiev, Breno Alencar Gonçalves, and Pedro Alessio. 2018. “Hackathons in the Formal Learning Process.” Pp. 248–53 in Proceedings of the 23rd Annual ACM Conference on Innovation and Technology in Computer Science Education – ITiCSE 2018. Larnaca, Cyprus: ACM Press.
- Holtzman, Mellisa, and Chadwick Menning. 2015. “Integrating Experiential Learning and Applied Sociology to Promote Student Learning and Faculty Research.” College Teaching 63(3):112–18. doi: 10.1080/87567555.2015.1019825.
- Kolb, Alice Y., and David A. Kolb. 2005. “Learning Styles and Learning Spaces: Enhancing Experiential Learning in Higher Education.” Academy of Management Learning & Education 4(2):193–212. doi: 10.5465/AMLE.2005.17268566.
- Moore, David Thornton. 2010. “Forms and Issues in Experiential Learning.” New Directions for Teaching & Learning 2010(124):3–13. doi: 10.1002/tl.415.
- Pedaste, Margus, Mario Mäeots, Leo A. Siiman, Ton de Jong, Siswa A. N. van Riesen, Ellen T. Kamp, Constantinos C. Manoli, Zacharias C. Zacharia, and Eleftheria Tsourlidaki. 2015. “Phases of Inquiry-Based Learning: Definitions and the Inquiry Cycle.” Educational Research Review 14:47–61. doi: 10.1016/j.edurev.2015.02.003.
- Richterich, Annika. 2019. “Hacking Events: Project Development Practices and Technology Use at Hackathons.” Convergence 25(5–6):1000–1026. doi: 10.1177/1354856517709405.
- Ring, Gail L., Chelsea Waugaman, and Bob Brackett. 2017. “The Value of Career EPortfolios on Job Applicant Performance: Using Data to Determine Effectiveness.” International Journal of EPortfolio 7(2):12.
- Strait, Jean R., Katherine Nordyke, and Andrew Furco. 2015. EService-Learning: Creating Experiential Learning and Civic Engagement Through Online and Hybrid Courses. Herndon, UNITED STATES: Stylus Publishing, LLC.
- Van Wart, Audra, Theresa C. O’Brien, Susi Varvayanis, Janet Alder, Jennifer Greenier, Rebekah L. Layton, C. Abigail Stayart, Inge Wefes, and Ashley E. Brady. 2020. “Applying Experiential Learning to Career Development Training for Biomedical Graduate Students and Postdocs: Perspectives on Program Development and Design.” Cbe-Life Sciences Education 19(3):19:es7. doi: 10.1187/cbe.19-12-0270.
- Wright, Mary C. 2000. “Getting More out of Less: The Benefits of Short-Term Experiential Learning in Undergraduate Sociology Courses.” Teaching Sociology 28(2):116–26. doi: 10.2307/1319259.